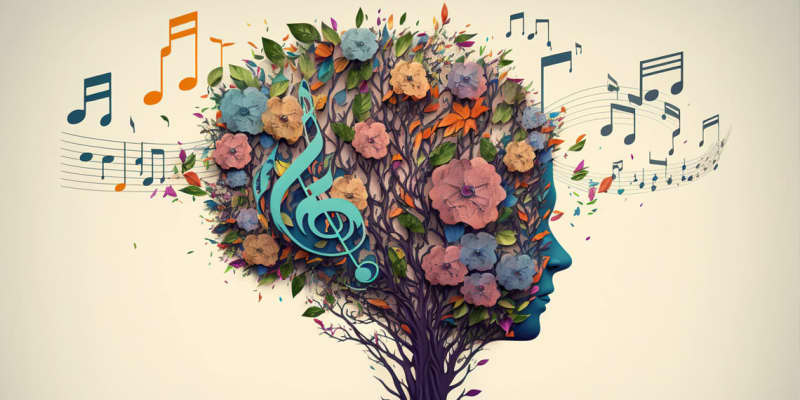
A team of scientists recently found they could predict which songs would become popular hits by measuring people’s brain activity while they listened to music and then analyzing the data using artificial intelligence. The models based on neurophysiologic measures provided significantly more accurate predictions compared to relying on people’s self-reported preferences. Their new study has been published in Frontiers in Artificial Intelligence.
Streaming services like Spotify and Pandora have invested in technologies to identify and introduce new music tailored to subscribers’ existing playlists. However, less than 4% of new songs become hits. Various methods have been used to predict hit music, including analyzing lyrics and social media mentions. But the predictive accuracy of these methods has been low.
The researchers behind the new study wanted to explore whether measuring neurophysiologic responses to music could help predict hit songs. Music has a profound effect on people’s emotions, and it activates multiple brain regions associated with emotion processing and long-term memory retrieval. The researchers believed that measuring peripheral neurophysiologic responses, such as cardiac signals associated with attention and emotional resonance, could capture the neural circuits involved in emotional responses to music.
“I had a meeting with a researcher at a music streaming service and he told me that 24,000 new songs are released daily worldwide and how his team struggles to determine which ones are likely to be hits,” explained study author Paul J. Zak, a professor at Claremont Graduate University, director of the Center for Neuroeconomics Studies, and author of “Immersion: The Science of the Extraordinary and the Source of Happiness.”
“My research had identified a neural pathway I have named ‘Immersion’ that appears to determine the value that the brain assigns to social-emotional experiences and I wondered if this neural activity could identify hit songs when they were first released. The streaming service sent me a set of newly released songs and the number of streams 3 months later.”
The study involved 33 participants who listened to 24 recent songs chosen by a streaming service. The participants’ neurophysiologic responses were measured using a commercial platform that captured signals associated with attention and emotional resonance. The researchers derived three variables from the neurophysiologic data: average immersion, peak immersion (the highest immersion moments during the song), and retreat (the lowest 20% of immersion moments).
Participants also provided ratings of their liking of each song, whether they would replay the song, recommend it to others, familiarity with the song, and whether they found it offensive. Market data, such as the number of song streams and online likes, were obtained from the streaming service.
The researchers found that by analyzing participants’ neurophysiologic responses to songs, they could accurately predict market outcomes, such as the number of song streams, with a small amount of data. This approach, called neuroforecasting, allows them to make predictions about the population based on the neural activity of a few individuals, without needing to measure the brain activity of a large number of people.
They compared the predictive accuracy of different statistical approaches and found that a linear statistical model was able to identify hit songs with a high success rate. However, when they applied machine learning techniques to the neurophysiologic data, the accuracy of correctly identifying hit songs increased to nearly 100%.
The study’s findings suggest that neurophysiologic measures, specifically measures of immersion and retreat during music listening, can accurately identify hit songs. Hits generally had higher immersion and produced less neurologic retreat compared to flops. Machine learning models, particularly a k-nearest neighbors algorithm, played a significant role in achieving high prediction accuracy.
“We showed that a linear model to predict hits using two neural variables was 69% accurate,” Zak told PsyPost. “This is not bad, but when we applied machine learning to these two variables, our predictive accuracy went to 97%. This occurred because the brain has inherent nonlinearity that machine learning models capture. Yet, the near perfect accuracy at predicting hits was quite surprising. No attributes of the songs or self-reported data were used in these predictions, only neurologic data.”
The researchers discovered that self-reported measures of liking a song were not predictive of whether it would become a hit.
“The conscious self-reported ‘liking’ of songs and a self-reported willingness to share them had zero predictive ability to identify hits,” Zak explained. “But, unconscious emotional brain activity can identify hit songs with very high accuracy even though this information is not open to our conscious awareness.”
“The big point is that our brain knows much more than we are consciously aware of. The reduction in the cost of wearable neurotechnologies, as used in this study, meaning that very soon everyone can have access to their unconscious emotional brain activity so we can learn more about ourselves and how we interact with our environment.”
The researchers acknowledge some limitations, such as the relatively small sample size and the use of synthetic data for training the machine learning model. Further research is needed to validate the model with larger song databases and external samples of songs to ensure its generalizability.
“We were only given 24 songs to measure and our sample skewed young so certainly more songs and a different population should be tested. But, given our high accuracy I am confident that our results will replicate. Our next step is to measure other types of entertainment to predict hits.”
“This study came about because I have actively chosen to interact with people who have hard problems they are trying to solve rather than spend every day in my lab or office. I think it is really important that scientists engage with the world in order to work on research that will be useful to society.”
The study, “Accurately predicting hit songs using neurophysiology and machine learning“, was authored by Sean H. Merritt, Kevin Gaffuri, and Paul J. Zak.