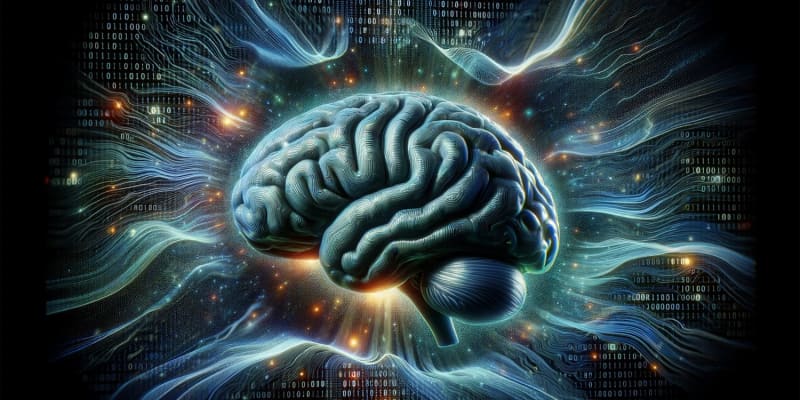
Researchers have developed an artificial intelligence model that successfully determines the sex of an individual based on brain activity scans with more than 90% accuracy. Published in the Proceedings of the National Academy of Sciences, this study sheds light on the long-debated topic of whether there are significant differences in brain activity between men and women. It suggests that recognizing these differences is crucial for understanding and treating neuropsychiatric conditions that disproportionately affect one sex over the other.
Previous research has hinted at sex playing a pivotal role in various stages of brain development, from early formation through adolescence and into aging. Moreover, there’s a notable disparity in the prevalence and manifestation of neuropsychiatric conditions between the sexes; for instance, women are more susceptible to depression, anxiety, and eating disorders, while men are more likely to develop autism, attention-deficit/hyperactivity disorder, and schizophrenia.
These conditions not only differ in frequency between sexes but also exhibit sex-specific clinical features and outcomes. The intricate relationship between sex and brain function necessitates a deeper understanding to enhance our approach to treating these conditions effectively.
However, despite substantial evidence pointing to anatomical differences in male and female brains, the impact of these differences on brain functionality and their translation into observable behavioral and cognitive variations have remained areas of contention and uncertainty. Addressing this gap in knowledge, the study sought to harness the capabilities of advanced artificial intelligence (AI) to explore the functional organization of the brain across sexes.
“A key motivation for this study is that sex plays a crucial role in human brain development, in aging, and in the manifestation of psychiatric and neurological disorders,” said Vinod Menon, a professor of psychiatry and behavioral sciences and director of the Stanford Cognitive and Systems Neuroscience Laboratory. “Identifying consistent and replicable sex differences in the healthy adult brain is a critical step toward a deeper understanding of sex-specific vulnerabilities in psychiatric and neurological disorders.”
The study involved the development and application of an end-to-end spatiotemporal deep neural network (stDNN) model, combined with an explainable artificial intelligence (XAI) framework, to analyze resting-state functional MRI (rsfMRI) data. The data were sourced from a large cohort of approximately 1,000 young adults aged 20 to 35 years, participating in the Human Connectome Project.
To ensure the reliability and interpretability of their findings, the researchers utilized the integrated gradients (IG) algorithm, a technique within the XAI framework, to identify and rank brain features or “fingerprints” that differentiate male from female brain organization. This approach provided a quantitative measure of the contribution of each brain feature to the model’s classification decision, enabling the identification of specific regions and networks underpinning sex differences in brain function.
The study also included rigorous cross-validation and consensus analysis to assess the replicability and stability of the identified sex differences across different data sessions and independent cohorts. Furthermore, to validate the robustness of their findings against potential confounding factors, the team conducted control analyses using different brain atlases, artifact reduction methods, and evaluations of head movement effects.
The study revealed specific brain regions and networks that contribute to the observed sex differences in functional brain organization. Notably, areas associated with the default mode network (DMN), including the posterior cingulate cortex, precuneus, and ventromedial prefrontal cortex, emerged as key discriminators between male and female brains.
This finding is particularly intriguing given the DMN’s role in self-referential thought and emotional processing, suggesting that men and women may engage these cognitive and affective processes differently.
Additionally, the analysis highlighted significant differences in the striatum and limbic networks, which are involved in reward sensitivity, reinforcement learning, and emotional regulation. These differences could have profound implications for understanding sex-specific vulnerabilities to psychiatric and neurological disorders, as well as for developing targeted interventions.
“This is a very strong piece of evidence that sex is a robust determinant of human brain organization,” Menon said.
The study also ventured into exploring the relationship between these sex-specific brain functional organizations and cognitive profiles. By analyzing behavioral data alongside brain imaging, the researchers identified distinct cognitive profiles that correlate with the unique brain features of males and females.
This aspect of the research sheds light on the behavioral relevance of the identified sex differences in brain function, suggesting potential pathways through which these differences might manifest in cognitive and emotional behaviors. The ability of the AI model to predict cognitive profiles based on brain function further emphasizes the practical significance of understanding sex differences in brain organization, not only for academic inquiry but also for clinical application.
“These models worked really well because we successfully separated brain patterns between sexes,” Menon said. “That tells me that overlooking sex differences in brain organization could lead us to miss key factors underlying neuropsychiatric disorders.”
However, the study includes some limitations. The focus on young adults limits the applicability of the findings across the lifespan, particularly in relation to developmental and aging processes. Additionally, the reliance on data from specific cohorts and imaging techniques may affect the generalizability of the results. Future research is encouraged to expand the demographic and methodological scope of the study, explore the underlying mechanisms of observed sex differences, and examine their implications for individual susceptibility to psychiatric and neurological disorders.
Menon said that their AI model is versatile enough to explore connections between virtually any facet of brain connectivity and various cognitive abilities or behaviors. He and his team intend to release their model for public use, allowing researchers worldwide to apply it to their investigations.
“Our AI models have very broad applicability,” Menon said. “A researcher could use our models to look for brain differences linked to learning impairments or social functioning differences, for instance — aspects we are keen to understand better to aid individuals in adapting to and surmounting these challenges.”
The study, “Deep learning models reveal replicable, generalizable, and behaviorally relevant sex differences in human functional brain organization,” was authored by Srikanth Ryali, Yuan Zhang, Carlo de los Angeles, Kaustubh Supekar, and Vinod Menon.