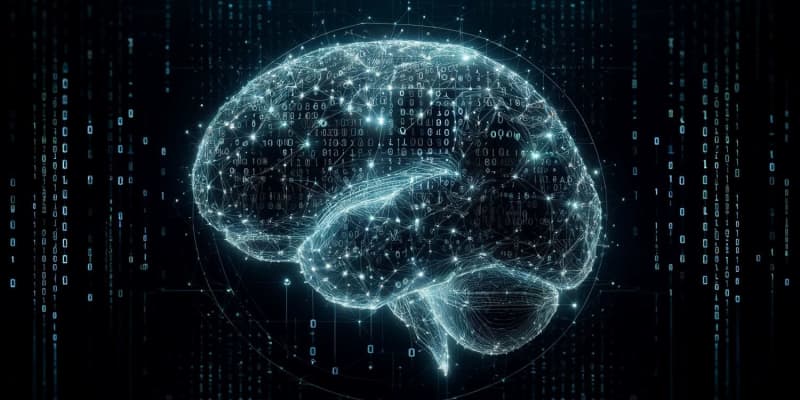
In the rapidly evolving field of artificial intelligence, a new study published in PLOS One has shed light on an unexpected capability of large language models like ChatGPT: their ability to mimic the cognitive and linguistic abilities of children. Researchers have found that these advanced AI systems can simulate lower levels of intelligence, specifically child-like language and understanding, particularly in tasks designed to test the theory of mind.
Large language models are advanced artificial intelligence systems designed to understand, generate, and interact using natural human language. These models are trained on a vast amount of text data, which enables them to produce remarkably human-like text, answer questions, write essays, translate languages, and even create poetry or code. The architecture of these models allows them to predict the next word in a sentence by considering the context provided by the words that precede it.
Theory of Mind is a psychological concept that refers to the ability to attribute mental states—beliefs, intents, desires, emotions, knowledge, etc.—to oneself and others and to understand that others have beliefs, desires, intentions, and perspectives that are different from one’s own. This capability is crucial for human interaction, as it enables individuals to predict and interpret the behavior of others, navigate social situations, and engage in empathetic and cooperative behaviors.
The researchers conducted their study to explore the extent to which large language models can simulate not just advanced cognitive abilities but also the more nuanced, developmental stages of human cognitive and linguistic capabilities, specifically those observed in children. This interest stems from the evolving understanding of AI’s capabilities and limitations.
“Thanks to psycholinguistics, we have a relatively comprehensive understanding of what children are capable of at various ages,” explained study author Anna Marklová of the Humboldt University of Berlin. “In particular, the Theory of Mind plays a significant role, as it explores the inner world of the child and is not easily emulated by observing simple statistical patterns.”
“We used this insight to determine whether large language models can pretend to be less capable than they are. In fact, this represents a practical application of concepts that have been discussed in psycholinguistics for decades.”
The researchers conducted 1,296 independent trials, employing GPT-3.5-turbo and GPT-4 to generate responses that would be analyzed for their linguistic complexity and accuracy in solving false-belief tasks. The core objective was to assess if these large language models could adjust their responses to reflect the developmental stages of language complexity and cognitive abilities typical of children aged between 1 and 6 years.
To assess linguistic complexity, the researchers employed two primary methods: measuring the response length and approximating the Kolmogorov complexity. Response length was chosen as a straightforward metric, operationalized by counting the number of letters in the text generated by the model in response to the prompts.
The Kolmogorov complexity, on the other hand, offers a more nuanced measure of linguistic complexity. It is defined as the minimum amount of information required to describe or reproduce a given string of text.
As the simulated age of the child persona increased, so did the complexity of the language used by the models. This trend was consistent across both GPT-3.5-turbo and GPT-4, indicating that these large language models possess an understanding of language development that allows them to approximate the linguistic capabilities of children at different ages.
The false-belief tasks chosen for this study were the change-of-location and unexpected-content tasks, both foundational in assessing a child’s development of Theory of Mind. As the name implies, these tasks test an individual’s ability to understand that another person can hold a belief that is false.
The change-of-location task involves a character, Maxi, who places an object (like a chocolate) in one location and leaves. While Maxi is gone, the object is moved to a different location. The task is to predict where Maxi will look for the object upon returning. Success in this task indicates an understanding that Maxi’s belief about the location of the object did not change, despite the actual relocation.
In the unexpected-content task, a container typically associated with a certain content (e.g., a candy box) is shown to contain something unexpected (e.g., pencils). The question then explores what a third party, unaware of the switch, would believe is inside the container. This task assesses the ability to understand that others’ beliefs can be based on false premises.
Both GPT-3.5-turbo and GPT-4 showed an ability to accurately respond to these false-belief scenarios, with performance improving as the age of the simulated child persona increased. This improvement aligns with the natural progression seen in children, where older children typically have a more developed Theory of Mind and are better at understanding that others may hold beliefs different from their own.
“Large language models are capable of feigning lower intelligence than they possess,” Marklová told PsyPost. “This implies that in the development of Artificial Superintelligence (ASI), we must be cautious not to demand that they emulate a human, and therefore limited, intelligence. Additionally, it suggests that we may underestimate their capabilities for an extended period, which is not a safe situation.”
An interesting finding was the occurrence of what the researchers termed “hyper-accuracy” in GPT-4’s responses to false-belief tasks, even at the youngest simulated ages. This phenomenon, where the model displayed a higher than expected understanding of ToM concepts, was attributed to the extensive training and reinforcement learning from human feedback (RLHF) that GPT-4 underwent.
RLHF is a training methodology that refines the model’s responses based on feedback from human evaluators, effectively teaching the model to generate more desirable outputs. This approach is part of the broader training and fine-tuning strategies employed to enhance the capabilities of AI systems.
“The effect of RLHF in the new model led to more adult-like responses even in very young personas,” Marklová explained. “It seems that the default setting of new models, i.e., they are ‘helpful assistants,’ adds certain constraints on the diversity of responses we as users can get from them.”
The study’s findings pave the way for several directions for future research. One key area involves further probing the limits of large language models (LLMs) in simulating cognitive and linguistic development stages across a wider array of tasks and contexts.
“We aim to triangulate psycholinguistic research with behavioral studies of large language models,” Marklová said.
Additionally, future studies could explore the implications of these simulations for practical applications, such as personalized learning tools or therapeutic AI that can adapt to the cognitive and emotional development stages of users.
“Our research aims to explore the potential of large language models, not assess if they are ‘good’ or ‘bad,” Marklová noted.
The study, “Large language models are able to downplay their cognitive abilities to fit the persona they simulate,” was authored by Jiří Milička, Anna Marklová, Klára VanSlambrouck, Eva Pospíšilová, Jana Šimsová, Samuel Harvan, and Ondřej Drobil.