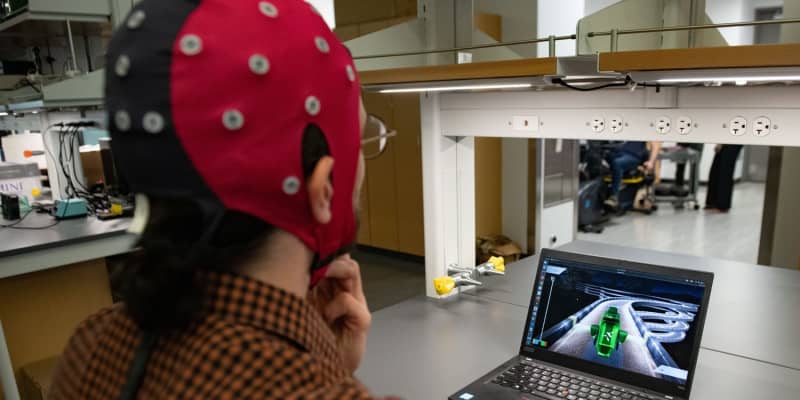
In a study by researchers at The University of Texas at Austin, a new type of brain-computer interface (BCI) has been developed that simplifies the use of such devices. This new technology allows users to control digital interfaces, like video games, directly with their minds without the lengthy setup previously required for each individual. This “one-size-fits-all” solution could significantly enhance the quality of life for people with motor disabilities by making the technology more accessible and easier to use.
The research was published in PNAS Nexus.
BCIs, which allow users to control devices using their brain signals, traditionally require each new user to undergo a calibration process to tailor the system to their unique neurological patterns. This calibration is not only labor-intensive but also requires the expertise of trained personnel, which limits the technology’s accessibility and scalability, particularly in clinical environments where quick adaptation from one patient to another is crucial.
The motivation for the study was to enhance the practicality and user-friendliness of BCIs, making them more accessible for people with motor disabilities. The researchers aimed to develop a system that could adapt to any user with minimal initial setup, thereby democratizing the use of such advanced technology. By simplifying the calibration process, the technology could be used more efficiently in various settings, ranging from medical facilities to home care, and even in consumer electronics for gaming and interaction.
The team at the University of Texas, led by Professor José del R. Millán, utilized a cap fitted with electrodes that measure the brain’s electrical signals. These signals are then decoded using machine learning algorithms that translate them into commands for digital tasks.
To test the adaptability and effectiveness of their new system, the researchers selected a sample of 18 healthy volunteers, none of whom had any motor impairments. These subjects were tasked with controlling two different digital interfaces: a simple bar-balancing game and a more complex car racing game.
The bar game was designed to be straightforward, requiring the subjects to balance the left and right sides of a digital bar, which served as an initial training tool for the decoder. The more challenging car racing game required subjects to execute a series of turns, mimicking the planning and execution needed in real-world driving scenarios.
The unique aspect of their experimental setup was the use of a machine learning model that could quickly adapt to each user’s brain activity. This model was initially trained using data from a single expert user, then adjusted to new users without the need for personalized calibration sessions. The system employed advanced machine learning algorithms to interpret EEG signals and refine its predictions through ongoing user interaction, effectively learning in real-time.
The researchers found that their system could successfully translate the EEG data into accurate commands for both the bar and the racing game, with participants able to control the interfaces effectively after minimal exposure to the system. This rapid adaptability was a significant advancement over traditional BCIs, which require lengthy and complex calibration for each new user.
“When we think about this in a clinical setting, this technology will make it so we won’t need a specialized team to do this calibration process, which is long and tedious,” said Satyam Kumar, a graduate student in the lab of José del R. Millán, a professor in the Cockrell School of Engineering’s Chandra Family Department of Electrical and Computer Engineering and Dell Medical School’s Department of Neurology. “It will be much faster to move from patient to patient.”
Moreover, the study demonstrated that the machine learning model was not only capable of adapting quickly but also improved its accuracy and responsiveness with use. This improvement suggested that the system was continuously learning and adjusting to the user’s specific brain patterns, thereby enhancing its effectiveness over time.
The ability of the BCI to operate efficiently across different tasks and users without individual recalibration opens up new possibilities for its application, particularly in environments where quick setup and ease of use are crucial, such as in clinical settings or home-based care for individuals with disabilities.
While the study’s results are promising, the technology was only tested on subjects without motor impairments. Future research will need to include individuals with disabilities to fully understand the interface’s effectiveness and practicality in the intended user population. Moreover, the current tasks tested were relatively simple. Future studies are expected to explore more complex applications and longer-term use to determine how well the interface supports continuous learning and adaptation.
Additionally, the research highlights the potential for using this technology in more dynamic settings, such as controlling wheelchairs or other assistive devices, which could significantly enhance mobility and independence for those with severe motor impairments.
“The point of this technology is to help people, help them in their everyday lives,” Millán said. “We’ll continue down this path wherever it takes us in the pursuit of helping people.”
The study, “Transfer learning promotes acquisition of individual BCI skills,” was authored by Satyam Kumar, Hussein Alawieh, Frigyes Samuel Racz, Rawan Fakhreddine, and José del R Millán.