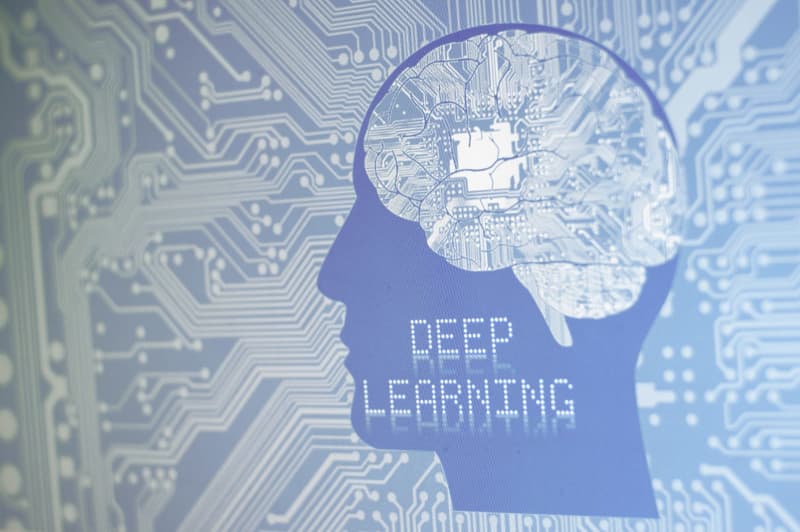
Language models have become a key factor when it comes to creating the most thorough and accurate artificial intelligence possible. The new model developed by Microsoft and Nvidia is said to feature about 530 billion parameters and to be capable of exceptional accuracy, especially in reading comprehension and complex sentence formation.
Nvidia and Microsoft's Megatron-Turing Natural Language Generation model (MT-NLG) marks a new record for a language model. According to the tech firms, their model is the most powerful to date. Thanks to its 530 billion parameters, it is able to outperform OpenAI's GPT-3 as well as Google's BRET. Specialized in natural language, it is able to understand texts, reason and make deductions to form a complete and precise sentence.
Language models are built around a statistical approach. While many methods exist, it is the n-gram model that is being used here. The learning phase enables analysis of a large quantity of texts to estimate the probabilities that a word will 'fit' correctly in a sentence. The probability of a word sequence is the product of the probabilities of the words previously used. By using probabilities, we can create perfectly grammatical sentences.
Biased algorithms still an issue
With 530 billion parameters, the MT-NLP model is particularly sophisticated. In the field of machine learning, parameters are often defined as the unit of measurement for machine performance. It has been repeatedly shown that models with a large number of parameters ultimately perform better, resulting in more accurate, nuanced language due to their large dataset. These models are capable of summarizing books and texts and even writing poems.
To train MT-NLG, Microsoft and Nvidia created their own dataset of about 270 billion "tokens" from English-language websites. In natural language, "tokens" are used to break up text into smaller chunks to better distribute information. The websites included academic sources such as Arxiv, Pubmed, educational websites such as Wikipedia or Github as well as news articles and even messages on social networks.
As always with language models, the main problem with widespread, public use is bias in the algorithms. The data used to train machine learning algorithms contain human stereotypes embedded in the texts. Gender, racial, physical and religious biases are widely present in these models. And it is particularly difficult to remove these problems.
For Microsoft and Nvidia, this is one of the main challenges with such a model. Both companies say that the use of MT-NLG "must ensure that proper measures are put in place to mitigate and minimize potential harm to users." Before fully benefiting from these revolutionary models, this issue needs to be tackled, and for the moment it seems far from resolved.