By Darren Parkin
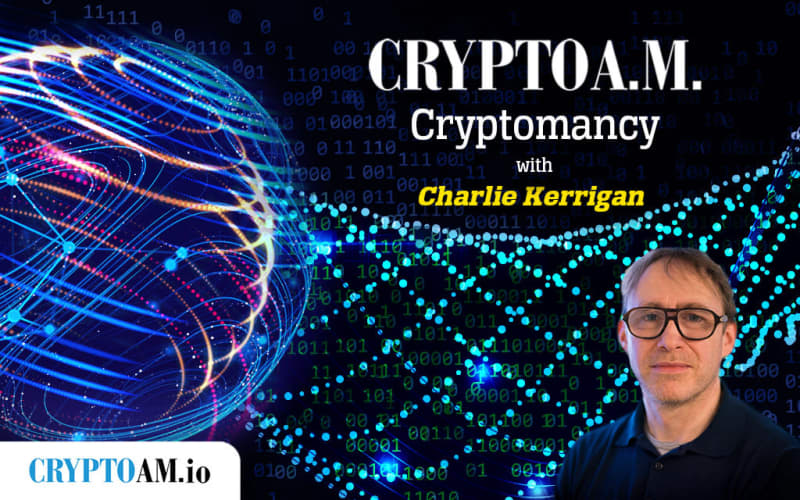
by Charles Kerrigan and Emre Kasim
The use of AI across all industries is proliferating. Automation is being adopted by businesses to replicate processes and decisions traditionally carried out by humans, allowing them to scale efficiently and remain competitive.
Despite these advantages, using AI can also introduce risks and high-profile harms. AI systems used in recruitment, policing and retail banking have been shown to be unreliable and unfair to women and those from traditionally marginalised communities.
Insurance is no different. AI is being used in assessing risk, fraud detection, underwriting, customer acquisition and targeting, as well as in customer service. Using AI to carry out these activities means that claims can be resolved in minutes, contracts can be drawn up rapidly, and quotes can be issued almost instantly. However, insurance policies are highly personalised and based on an individual’s demographic and history.
This process brings concerns over how reliable and fair such systems will be.
For example, in the context of health insurance, AI Is being used to predict instances of early disease onset, how likely hospitalisations are, and how likely patients are to take their prescribed medication.
Based on these outcomes, health insurers can then prioritise patients who need interventions. However, these algorithms can be affected by factors such as lack of representation of minority subgroups in the training data, systemic racism, and differences in the accuracy of models for different subgroups – particularly since diseases can present differently in different races. This can result in some subgroups not receiving the mental attention that they need if bias is not tested for and mitigated in the algorithms used by health insurance providers.
Bias has also been discovered in the algorithms used to determine car insurance premiums in the US. Residents of predominantly minority areas being given higher quotes than those living in non-minority areas and which are associated with similar levels of risk.
This difference in premiums was greatest in the states of California, Texas, and Missouri, with residents of minority areas being charged around 10% more than those in non-minority areas.
These findings demonstrate that even when insurers do not directly use race as a predictor in their algorithms, zip code can still be used as a proxy, and can result in minority subgroups being discriminated against by insurers.
In light of these risks and the fact that insurance is a high-risk context, it is important that the danger of AI bias is identified and steps taken to mitigate them. This is particularly important as insurance can be a legal requirement and can have financial and health-related implications for (prospective) policy holders.
While some companies will opt to do this voluntarily, AI risk management can also be required by law. In the context of insurance, Colorado has passed legislation requiring insurers to commission third-party bias audits of their algorithms and datasets. The purpose is to identify discrimination based on protected characteristics such as race, ethnicity, sex, or gender.
Under this legislation, insurers will also be required to adopt a risk management framework to continually monitor their models and data for bias, or discriminatory outcomes, and provide an explanation of how predictive models and data are being used to make insurance-related decisions.
The aim of this legislation is to make the use of AI in insurance safer and fairer for users, particularly those from marginalised subgroups, AI in insurance needs to be safe and fair, and AI risk management will be vital for achieving this and preventing further instances of harm.
Charles Kerrigan is a FinTech partner with law firm CMS, and Emre Kasim is COO at Holistic AI
The post AI in insurance needs to be safe and fair appeared first on CityAM.